The Rise of Autonomous AI Agents: Debundling the Market Landscape
.png)
Introduction
The world of AI is evolving at a breathtaking pace. The introduction of Autonomous AI Agents (Agents) is a testament to how this landscape is maturing.
Agents are systems that are capable of perceiving their environment, making decisions or taking actions, and achieving specific goals or objectives.
The Agents Market is estimated to grow from USD 5B in 2023 to USD 29B by 2028, at a CAGR of 43%. Recent interest in the area has sparked the term “Agents are the tulip’s AI summer”.

They are the next big thing.
“Agents are the next wave — not just in tech but in business at large. I predict that within 10 years, there will be multiple billion-dollar companies run entirely by autonomous agents” Ben Parr, Co-founder, Octane AI
Agents provide a step change in capabilities vs LLMs
Agents’ abilities to execute extremely complex tasks and problem solve, unlocks many new use cases previously unrealisable by LLMs alone.
An example of this for a job to be done is in EdTech: the move from 1:many to 1:1 tuition, where the tutor Agent is able to continuously adapt and support the child’s learning experience and deliver an extremely personalised course based on a child’s needs (Cornell University).
There are two popularised frameworks for Agents
These are SWYX’s framework, which parallels agents to the human brain, and Lillian Wang’s recent framework, which deep-dives into sub-components across memory, tooling, planning and actioning.

The ability to decompose tasks, adapt, interact and store memory is key to both of these. These tasks repeat in a loop:
- Set objectives and select tasks — programmed with objectives to be achieved and chunking these into tasks
- Adapt to new stimuli — learn from their experiences and adapt behaviour to environments. Performance improves with time
- Interact and execute — the ability to interact with multiple stimuli, including other agents, tooling or human users e.g., browsing the internet
- Perceive responses — process and interpret data to make informed decisions
- Store long and short-term memory — store longitudinal memory
Thesis: Debundling of the “God Mode Agent Stack” and Agents as a Service
Demand for Agents will grow as they save humans the scarce resources of time and money. Here are our two forward-looking theses in the areas, driven by different factors:
Thesis 1: Unbundling of the God AI Agent stack
Ultimately, the “God Mode AI Agent Stack”, which alludes to the concepts of one AI ruling all through bringing together multiple tools / APIs across various use cases, will be debundled. Our thesis is this landscape will be fragmented. The thesis behind this is the concept of multiple “AI fleets”. A winner-takes-all approach to this market may be viewed as anti-competitive. The rise and power of multi-agent systems (MAS) has also driven this forwards (Appypie).
Thesis 2: Agents as a Service
The infrastructure layer that enables the deployment of Agents is still nascent, with most Agent frameworks, such as BabyAGI, being experimental open-source projects. As the demand for Agents grows, so will the need to formalise these foundations. Each sub-segment will be productized (made into an app or API) over time. Humans will eventually be able to create or hire Agents as a product. For example, the AI Autonomous worker Katherine, “she works for Visla and bids for work on up work as a copywriter. She has been successful in competing for jobs” (Theeuf).
The emerging Autonomous AI Agent Market Landscape
Although this is a nascent area, we have identified three key layers to the Autonomous AI Agent Market that align with the way we are looking at our investment theses.

Breaking down each component of the map, in order of maturity:
AgentOps
There are seven core components to this. These combine to form “frameworks”, which will be templatized and eventually listed on an Agent Ops like marketplace for distribution. Early POCs include Teenage-AGI, Baby-AGI, AutoAGI.
- 🧠Intelligence — the “brains” of the agent, powered by LLMs responsible for task creation, planning and context. They understand and generate natural language, possess vast world knowledge, and can learn. LLMs are used via API or Open Source. More progressed examples involve HuggingGPT, Falcon, and GPT-4. Domain-specific DaaS startups and model hubs also fall into this category e.g., Sahha provides an API for mental health data. Distribution advantage, costs, community moats and model quality will be key winning.
- 📃Agent memory — acquiring, storing, retaining and retrieving data. This is divided into short, long term and sensory memory (read more here). The proliferation of vector databases and embedding frameworks are key to this. Players include Pinecone and Chroma, as well as Text to SQL startups like Perplexity AI which have integration advantages.
- 🔌Tools and plugins — create, modify and utilise external objects to do things that go beyond LLM limits. External tools can significantly extend the model capabilities e.g., browser scanning and desktop enablement. These utilities are predominantly in prompts and skill libraries at the moment. SLAPA is a self-learning API system that is an example of early productization. Relevance AI is another fast-moving player in this field differentiating on UX and the ability to create “chains of Agents easily with low code”. GPT is also creating plugin marketplaces. It will be interesting to see more platform agnostic platforms play here and those that are able to horizontally integrate.
- 👨👩👧Multi-agent communication schemas — agents made from a mixture of AgentOps will need services that enable them to interact in the most optimal way possible. This allows for the concept of multi-agent learning, reflection and interpretation. These exist predominantly as prompting techniques at the moment. For example, Chain of Thoughts prompting Wei et al (2022) and Reflexion (Shinn & Labash 2023). We predict this segment will mature and players which can productize these schemas e.g., in Drone Reforestation
- 🔮 Multi-agent playgrounds and protocols — the unavoidable question of ‘what communication protocol should be applied between these agent networks’ will be answered here (George, founder of Ayala). The need for a player which is the equivalent to If This Then That, but for Agents, is clear. Experiments in this have been locally hosted as POCs so far E.g., CAMEL, which has three Agent interaction. For example, a trading bot: the user Agent is a stock trader, the AI assistant is a py coder and the task-specific agent acts as a manager. Others include PumaMart and SIMs Gen Agents. E2B is a rising player in this space
- 👮♂️ Monitoring, security and budgetary — across intelligence, memory, tools and plugins, communication and protocols, safety and security should be monitored. Monetary guardrails should be inforced too. It will be interesting to see whether monitoring this at the tooling level or Agent level will be most impactful (still an open question). Nevertheless, players who can optimise Agents through monitoring (data lineage for errors), security and budget across multiple platforms will win.
- 🤝AgentOps marketplaces — HuggingFace has traditionally been the predominant player in model distribution. There is an opportunity to create a similar product for Agents, similar to what Smol-ai Developer does, where it will be an easy UX for AI Engineers to pick the optimal Agent infrastructure for tasks.
Applications
Agents will “become products”. As the landscape matures, we will see many of these being productized and monetized either through closed or open-source models (currently this sits as 50% split with most close-sourced models being targeted to more niche use cases — e2b analysis)
- 🧑 General purpose — These provide an unprecedented level of data-driven intelligence and democratise this intelligence for individual users. These will be centered around the framework of “jobs to be done”. Virality, the ability to establish distribution advantage, and playing into more “niche” use cases defensible from big tech will be key to winning. The main use cases at the moment are for personal coders e.g., GitWit, GPT-Engineer. The number of players in personal productivity and personal EAs is also growing Embra AI (MAC personal Assistant),Dust (productivity assistant),
- 🏢 Business industry (virtual workforce) — these are verticalized use cases fine-tuned with context-specific business rules or data. For employers, this enables significant cost reduction and performance uplift (reduced FTE count, more discussion here). We expect existing players who move downstream into AgentOps to have an advantage in this market. Their tech stack is more defensible. However, incumbents which build agilely (see GenAI investment thesis) may also win. This demand is growing fast, for example, Curious Thing AI are finding “Global customers wanting to hire Lucy, our voice AI suppoer bot” (David McKeague). Early movers include YC’s Flint, (EdTech), BrainFish (Sales) and Dialogue (design).
Services
- 🚀 Build your own Agent — we are seeing an influx of players in this category. These are low or no-code platforms enable any enterprise or consumer user to easily create and deploy Agents. To differentiate, UX, customer support and the ability to tailor usability for specific use cases (e.g., B2B, B2C, industries) will be key to winning. A key hurdle for these will be customer retention (LTV:CAC), these platforms will move from traction at all costs to retention at all costs. Integration and user experience e.g., support, will be key to increasing stickiness. Players include Relevance AI, XpressAI, SuperAgentAI and AgentRunnerAI.
- 💸Agent marketplaces — as the landscape explodes, apps will be listed on marketplaces for distribution. Our thesis is that this will be particularly true for B2C cases, where B2B will most likely be more direct (Google is the marketplace almost for B2B SaaS companies and we expect Apps to be similar). We are seeing existing hubs such as GitHub and Fiverr (surprisingly early adopters) beginning to play this role. NexusGPT is disrupting this with a freelance Agent marketplace and MindOS with broader focus. We may see “bundles” or fleets of AI workers specified to industries or functional areas sold as products in the future.
- 🎮Multi-agent monitoring — the control room engine for multiple agents is an emerging open category to be disrupted. There are use cases for both the enterprise and individual levels. The ability to build integrations and APIs in a platform agnostic way will be pivotal to winning. Although nascent, Alphakit.AI is a first mover in this space — allowing the monitoring of personal agents on the mobile phone.
Five predictions we are excited about
We are excited about opportunities that will provide long-term value and be deeply embedded in the Agent technology stack. Here are our top 5 predictions:
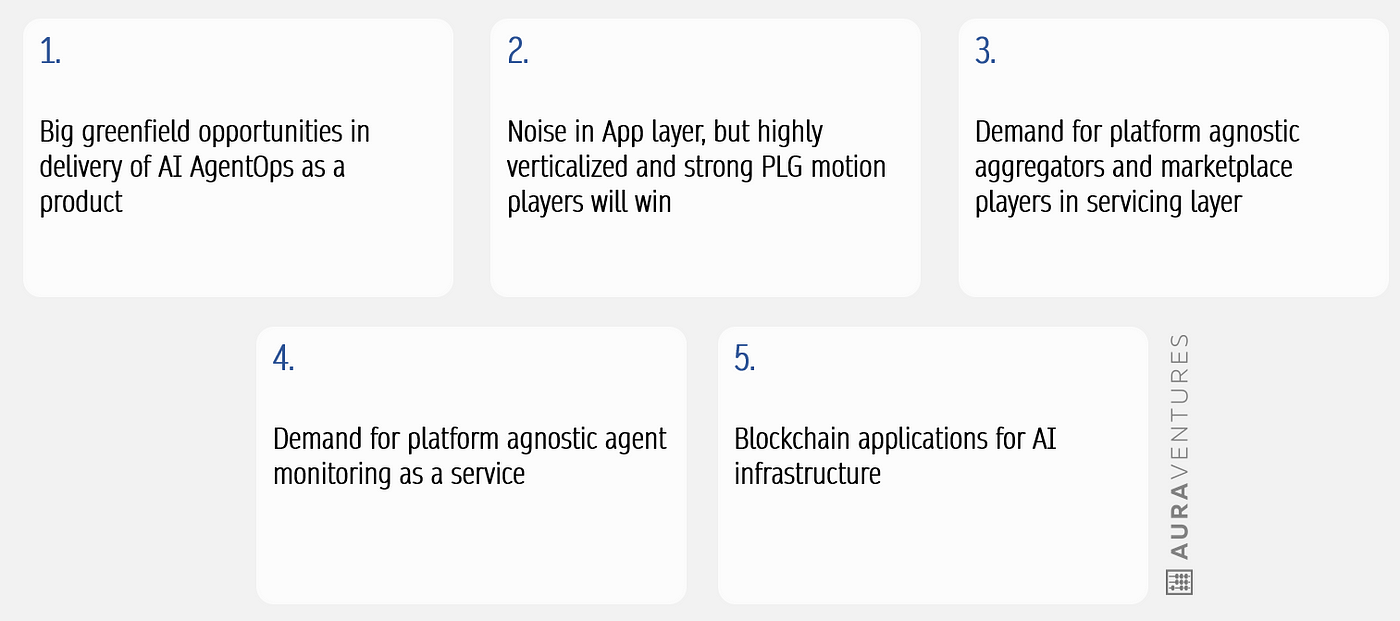
- White spaces in the delivery of AgentOps. This is essentially the productization of the AI Engineer stack. Current layers of AgentOps are still nascent, and mainly open source experiments. This is particularly true for multiagent protocols, communication schemas and monitoring / security. For the first two, we believe there is opportunity for incumbents with distribution and superior user experience to win. The market will be winner takes most, so players which can reach network effects or that easily integrate will win. It will be interesting to observe how verticalized industries and existing platform ecosystems compete e.g., OpenAI vs Google. Particularly if one player vertically moves away from traditional “picks and shovels’’ mechanisms to further build more into the nascent spaces…or if this space will be left to incumbents. We are excited for the opportunity in this fast evolving area.
- There will be noise in the application layer, and players who are highly verticalized or have strong PLG notions will win. The rapid self-learning mechanism of agent “native” startups will allow them to disrupt traditional SaaS markets. The phenomenon of the “solopreneur” drives this uncanny opportunity. Opportunities we are excited about are the combination of this with “intelligence” and weave together bespoke infra to execute targeted objectives. We believe there are two ways to “win” in this market. The first, is through verticalized industry used cases where Agents are embedded into workflows. For example, FinTechs are adopting a version of FinGPT and combining this with internal IP data. The second are API “Agents as a Service” plays. Startups will be able to drive a wedge as usage will become “table stakes” in driving efficiency and accuracy. This field will be driven by product lead growth. Existing players such as Sahha who are currently DaaS APIs will advance to AaaS (Agents as a Service). Gurleen, Sahha’s Head of Neuro states: “Sahha’s focus on building contextualised data moats will be key to unlocking the concept of white labelled personalised Doctors”. David states that tech IP will be key for both areas. “Single machine LLMs are an important trend for this. Finding new efficient ways of tuning and training will be critical” (Curious Thing AI).
- There will be a need for aggregators and marketplace players. This is pegged on the thesis of the debundling market. They will reach network effects sooner and given the nature of Agents also drive defensibility flywheels as more users interact with Agents. Early adoption will occur in marketplaces for marketing, designers and sales agents. Hence, a key opportunity is in the Gig Economy. SLAPA is a another great early example of such an aggregator. Those who have tailored agents, which have “product hunt” like capabilities, easily integrate or who are able to build strong community moats will win.
- Demand for platform agnostic Agent monitoring. A new white space will emerge in agent platforming and monitoring. This thesis is driven by fragmentation and demand for AI regulation. Startups who enable the tracing of “data” lineage will be key e.g., where agents are not working. Startups which are able to hold multiple integrations, gain unique distribution advantage, build community moats and with superior UX will win in this field. This could be a step towards “AI immune systems” as a whole new class of startups (more below).
- Proliferation of blockchain applications for AI infrastructure — This will particularly be true and may be a great enabler of advancements in the three factors mentioned below. Our thesis is that to reduce the risk of “scalability” and ethics, an approach similar to “DeFi” towards the concept of “DeAgents” will take place. Blockchain can provide a decentralized, transparent, and secure platform for developers to share data, code, and resources (HackerNoon). Players who create tailored solutions which have easy onboarding ramp ups and easily integrate will be able to succeed here.
Maturity of trust, tech and policy will determine pace of adoption
A trifecta of three factors (trust, tech and policy) maturing will define the pace for the move from AI co-pilots augmenting decision, to Agents for task execution to more fully fleshed Agent fleets — and moving toward “primitive AGI” (Sigi Chen).
Forecasting the progress of AI agents requires an evaluation of their added value and error tolerance. The more the value-add and the higher the error tolerance, the quicker we can expect AI agents to become mainstream in an industry or function — George, Founder of Ayala
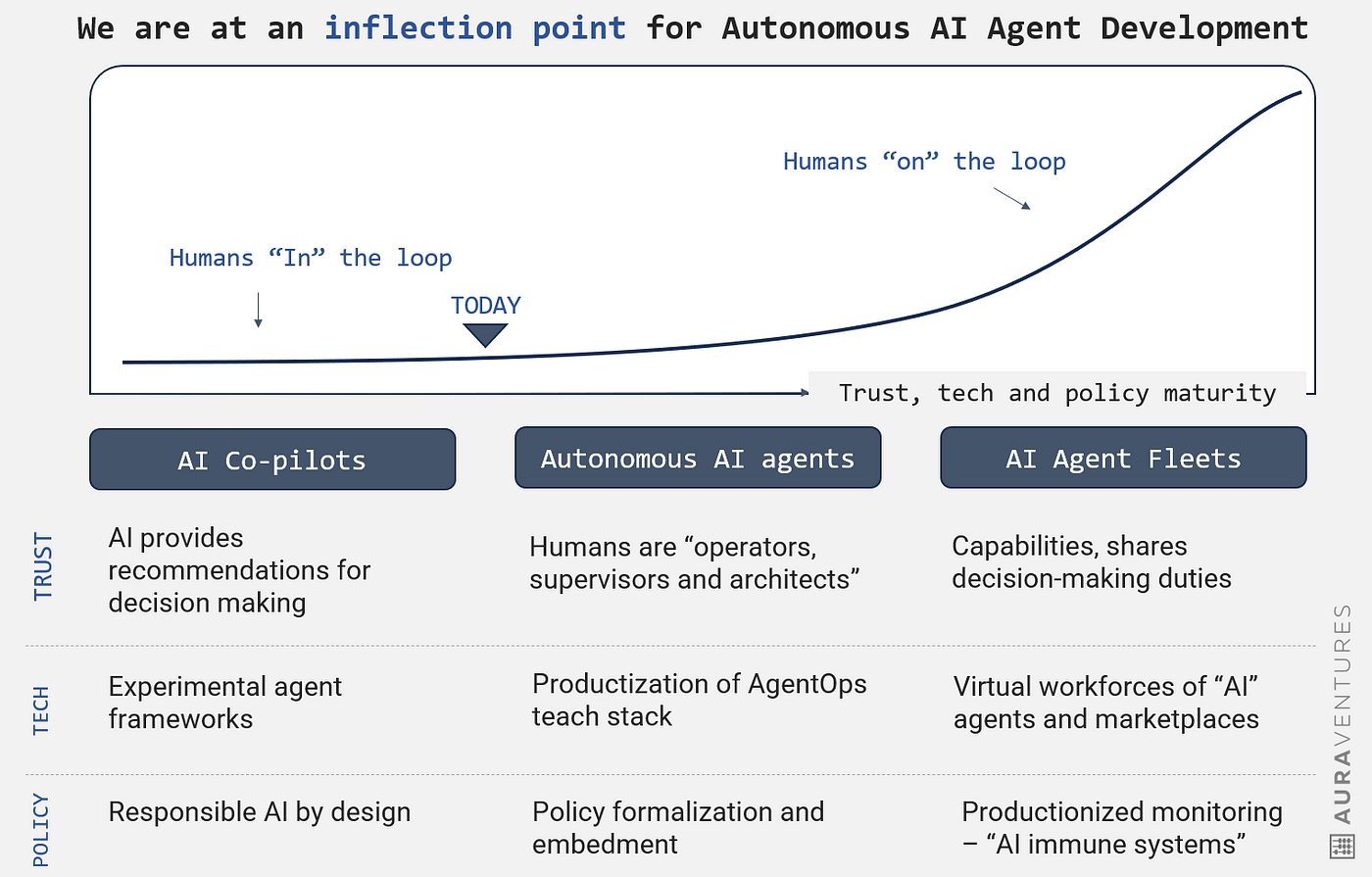
Trifecta deep-dive
- Trust: Despite AI advancements, humans remain vital for complex tasks. Finding the right balance between AI autonomy and human supervision is crucial. Trust in AI will grow, but the pace of this is unknown. The transition from read to write will be a big hurdle to overcome. E.g., discussion in the health field. There are two schools of thoughts around this at the moment: The Jobs School: AI Agents that augment your agency, as “bicycles for your mind” and the Zuck School: AI Algorithms that replace your agency, hijacking your mind. Yohei, the creator of BabyAGI states: “The future of autonomous agents looks like everybody becoming a manager”.
- Technical developments: There are two buckets of constraints from the technical perspective. The first is the current cost of running agents, who interact across multiple systems which are still maturing is expected to be relatively high. There is also a hypothesis that an “AI winter” is coming, with platforms such as Reddit and Twitter closing and beginning to charge for APIs. We expect this cost to be passed up the Agent stack. Theeuf states that “an AI winter may occur within the open source community, and this will have implications on the monopolistic behaviour ”. Secondly, from the technical perspective, there is finite context length for API to call context and responses, long -term planning, task decomposition errors and reliability of NLP interfaces (hallucinations).
- Policy, regulatory and legal advancements: Regulatory and legal policies and reforms will remain front of mind, both specific to Autonomous Agents and Generative AI. Being responsible AI by design should be a core consideration for startups (read more Responsible AI). Emergencies such as ChaosGPT are triggering calls to action. Recent Legal Theories in the area bring to light important, unanswered questions such as what legal status Autonomous Agents should have given ownership and copyright rules (source). These questions are still unanswered and without accelerated development in this field, AI agent deployment may be halted. Overtime, the concept of “AI immune systems” which can identify “bad actors” in the ecosystem may prevail, Prof Bedigo references this here.
Conclusion
One of my favourite founders and friends, Nilu from Stori (Gen AI gaming startup) summarises this candidly: “Right now the biggest use of AI agents is wasting your OpenAI bill…but this will change in the near future, AI Agents will become OP in abilities to execute complex, higher order tasks”.
So, which opportunity are you excited about, or maybe don’t think will be realised? I would love to hear your thoughts in the comments.
Thank you for reading. If any of these resonated with you please feel free to reach out.
Thank you to the AI experts, startups and industry experts for your inputs on this
Sources and further reading
- Emergency Pod: OpenAI’s new Functions API, up to 75% Price Drop, 4x Context Length (w/ Simon Willison, Riley Goodside, Roie Schwaber-Cohen, Joshua Lochner, Stefania Druga, Eric Elliott, Mayo Oshin) (latent.space)
- A Legal Theory for Autonomous Artificial Agents (umich.edu)
- The Anatomy of Autonomy: Why Agents are the next AI Killer App after ChatGPT (latent.space)
- Reflecting on Reflexion — by Noah Shinn and Ashwin Gopinath (substack.com)
- The Complete Beginners Guide To Autonomous Agents (mattprd.com)
- Software is Eating the World… but will Generative AI Eat it? | by Annie Liao | Aura Ventures | Medium
- AI Model Training Costs Are Expected to Rise from $100 Million to $500 Million by 2030 | Metaverse Post (mpost.io)
- The Anatomy of Autonomy: Why Agents are the next AI Killer App after ChatGPT (latent.space)
- 137 emergent abilities of large language models — Jason Wei
- GitHub — e2b-dev/awesome-ai-agents: A list of AI autonomous agents
- Why Autonomous AI Agents Are Silicon Valley’s Latest Obsession (businessinsider.com)
- ChatGPT, Next Level: Meet 10 Autonomous AI Agents: Auto-GPT, BabyAGI, AgentGPT, Microsoft Jarvis, ChaosGPT & friends | by Maximilian Vogel | The Generator | Medium
- Disruptive AI Tech: Know About The New Era Of Autonomous Agents (indiatimes.com)